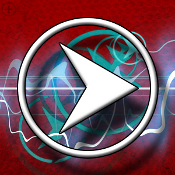
O'Reilly Data Show Podcast
Summary: The O'Reilly Data Show Podcast explores the opportunities and techniques driving big data, data science, and AI.
- Visit Website
- RSS
- Artist: O'Reilly Media
- Copyright: © 2020 O'Reilly Media
Podcasts:
In this episode of the Data Show, I spoke with Rhea Liu, analyst at China Tech Insights, a new research firm that is part of Tencent’s Online Media Group. If there’s one place where AI and machine learning are discussed even more than the San Francisco Bay Area, that would be China. Each time I go to China, there are new applications that weren’t widely available just the year before. This year, it was impossible to miss bike sharing, mobile payments seemed to be accepted everywhere, and people kept pointing out nascent applications of computer vision (facial recognition) to identity management and retail (unmanned stores).
In this episode of the Data Show, I spoke with Bruno Fernandez-Ruiz, co-founder and CTO of Nexar. We first met when he was leading Yahoo! technical teams charged with delivering a variety of large-scale, real-time data products. His new company is helping build out critical infrastructure for the emerging transportation sector. While some question whether V2X communication is necessary to get to fully autonomous vehicles, Nexar is already paving the way by demonstrating how a vehicle-to-vehicle (V2V) communication network can be built efficiently. As Fernandez-Ruiz points out, there are many applications for such a V2V network (safety being the most obvious one). I’m particularly fascinated by what such a network, and the accompanying data, opens up for future, smarter cities. As I pointed out in a post on continuous learning, simulations are an important component of training AI applications. It seems reasonable to expect that the data sets collected by V2V networks will be useful for smart city planners of the future. Here are some highlights from our conversation: The many applications of a vehicle-to-vehicle network Imagine if every vehicle on the road was equipped with a transponder that allowed it to connect to a network and say: ‘Hey, here I am. This is where I’m going. This is how fast I’ve gone. This is where I’ve been in the last 10 seconds. This is where I think I’m going to be in the next 10 seconds.’ Now imagine you were sharing that with all the vehicles around you so all these vehicles can predict, react, and even proact how they should behave on the road. You can start solving for safety and for traffic congestion. You start solving for utilization of the road, for pollution, and many other problems. … That’s kind of the vision. I think with autonomous vehicles on the road, this will be even more important. You’ll have humans sharing the road with them—then, how does this mix of human and autonomous vehicles talk to each other? … So, we’re trying to just build that vehicle-to-vehicle network. You can call it ‘ground traffic control.’ It’s similar to what happened in the air when radar and beaconing technology became available, and people said, ‘Well, we should probably connect to these things to be able to know where the planes are and tell them where to go.’ Redundancy and safety There are many situations in which other autonomous technologies actually may fail, and I think that’s where vehicle-to-vehicle communication becomes both a necessary technology for the true future of complete automation. It’s also a redundant system for when that camera, or for when that radar, when whatever other sensors in the car fail. Related resources: “Creating autonomous vehicle systems“: Understanding AV technologies and how to integrate them Cars that coordinate with people: 2017 AI Conference keynote by Anca Dragan “How big data and AI will reshape the automotive industry“ “How intelligent data platforms are powering smart cities“ “Why continuous learning is key to AI“: A look ahead at the tools and methods for learning from sparse feedback.
In this episode of the Data Show, I spoke with Bruno Fernandez-Ruiz, co-founder and CTO of Nexar. We first met when he was leading Yahoo! technical teams charged with delivering a variety of large-scale, real-time data products. His new company is helping build out critical infrastructure for the emerging transportation sector.
In this episode of the Data Show, I spoke with Carme Artigas, co-founder and CEO of Synergic Partners (a Telefonica company). As more companies adopt big data technologies and techniques, it’s useful to remember that the end goal is to extract information and insight. In fact, as with any collection of tools and technologies, the main challenge is identifying and prioritizing use cases. As Artigas describes, one can categorize use cases for big data into the following types: Improve decision-making or operational efficiency Generate new or additional revenue Predict or prevent fraud (forecasting or minimizing risks) Artigas has spent many years helping large organizations develop best practices for how to use data and analytics. We discussed some of the key challenges faced by organizations that wish to adopt big data technologies, centers of excellence for analytics, and AI in the enterprise. Here are some highlights from our conversation: Adopting big data analytics: Remaining key challenges For me, the first challenge is that there’s a lack of skills across organizations. We know there’s a global shortage of analytic talent, so it’s not only challenging for a company to acquire the right talent, but also to make sure that this talent is accessible across the organization. It’s usually concentrated in some departments, and it’s very difficult to leverage those skills for the good of the entire organization. The second challenge I see is lack of standards and lack of governance. You might find that every single data science team uses their own libraries or their own version of code or their own software tools. They are thinking about the benefit for a particular use case, and the best tools and the best models for that particular use case. But this compartmentalized approach cannot scale up; having a variety of versions of libraries and tools make it very, very difficult to industrialize and implement global solutions. Finally, new skills you need to develop are not only on the technical side—they are mostly on the business side. Decision-makers need to make decisions in different ways. They need to make decisions based on data, based on facts. Center of excellence for analytics The analytics center of excellence is a team of business and technical people that can be internal, external, and even crowd sourced. They have some centralized capabilities and also some distributed capabilities and resources, creating a common (online) workspace where they share methodologies, tools, models, and techniques. The objective is to gain efficiency and be able to implement initiatives across to the different business units. We have two main components of these centers of excellence: the business transformation unit and the deployment units. The business transformation unit (BTU) is the primary link of the center of excellence with the underlying business. We create ambassadors, and these ambassadors, who are part of the BTU, are responsible for identifying and prioritizing all business use cases. Then they connect with the deployment units—which we call cells—and these cells can grow organically during a project. So, first of all, the center of excellence must be connected with business. … We also create a centralized function called the ‘core team’ and an expansion unit called the ‘extended team.’ We have a few types of cells: the analytical cells, the operational cells, and the data utilization cells. So, it’s a way of concentrating the resources, gaining operational efficiency, having a center of know-how transferred to the rest of the organization, and ensuring best practices and methodologies. … A center of excellence is not a physical place. The center of excellence is a network of people who can be distributed in different geographies. Related resources: “The stages of enterprise IoT adoption“: Teresa Tung on building a business case for the Internet of Things “Data go
In this episode of the Data Show, I spoke with Carme Artigas, co-founder and CEO of Synergic Partners (a Telefonica company). As more companies adopt big data technologies and techniques, it’s useful to remember that the end goal is to extract information and insight. In fact, as with any collection of tools and technologies, the main challenge is identifying and prioritizing use cases.
In this episode of the Data Show, we look back to a recent conversation I had at the Spark Summit in San Francisco with Ion Stoica (UC Berkeley professor and executive chairman of Databricks) and Matei Zaharia (assistant professor at Stanford and chief technologist of Databricks). Stoica and Zaharia were core members of UC Berkeley’s AMPLab, which originated Apache Spark, Apache Mesos, and Alluxio. We began our conversation by discussing recent academic research that would be of interest to the Apache Spark community (Stoica leads the RISE Lab at UC Berkeley, Zaharia is part of Stanford’s DAWN Project). The bulk of our conversation centered around machine learning. Like many in the audience, I was first attracted to Spark because it simultaneously allowed me to scale machine learning algorithms to large data sets while providing reasonable latency. Here is a partial list of the items we discussed: The current state of machine learning in Spark. Given that a lot of innovation has taken place outside the Spark community (e.g., scikit-learn, TensorFlow, XGBoost), we discussed the role of Spark ML moving forward. The plan to make it easier to integrate advanced analytics libraries that aren’t “textbook machine learning,” like NLP, time series analysis, and graph analysis into Spark and Spark ML pipelines. Some upcoming projects from Berkeley and Stanford that target AI applications (including newer systems that provide lower latency, higher throughput). Recent Berkeley and Stanford projects that address two key bottlenecks in machine learning—lack of training data, and deploying and monitoring models in production. [Full disclosure: I am an advisor to Databricks.] Related resources: Spark: The Definitive Guide Advanced Analytics with Spark High-performance Spark Learning Path: Get Started with Natural Language Processing Using Python, Spark, and Scala Learning Path: Getting Up and Running with Apache Spark “The current state of applied data science”
In this episode of the Data Show, we look back to a recent conversation I had at the Spark Summit in San Francisco with Ion Stoica (UC Berkeley professor and executive chairman of Databricks) and Matei Zaharia (assistant professor at Stanford and chief technologist of Databricks). Stoica and Zaharia were core members of UC Berkeley’s AMPLab, which originated Apache Spark, Apache Mesos, and Alluxio.
In this episode of the Data Show, I spoke with Ken Stanley, founding member of Uber AI Labs and associate professor at the University of Central Florida. Stanley is an AI researcher and a leading pioneer in the field of neuroevolution—a method for evolving and learning neural networks through evolutionary algorithms. In a recent survey article, Stanley went through the history of neuroevolution and listed recent developments, including its applications to reinforcement learning problems. Stanley is also the co-author of a book entitled Why Greatness Cannot Be Planned: The Myth of the Objective—a book I’ve been recommending to anyone interested in innovation, public policy, and management. Inspired by Stanley’s research in neuroevolution (into topics like novelty search and open endedness), the book is filled with examples of how notions first uncovered in the field of AI can be applied to many other disciplines and domains. The book closes with a case study that hits closer to home—the current state of research in AI. One can think of machine learning and AI as a search for ever better algorithms and models. Stanley points out that gatekeepers (editors of research journals, conference organizers, and others) impose two objectives that researchers must meet before their work gets accepted or disseminated: (1) empirical: their work should beat incumbent methods on some benchmark task, and (2) theoretical: proposed new algorithms are better if they can be proven to have desirable properties. Stanley argues this means that interesting work (“stepping stones”) that fail to meet either of these criteria fall by the wayside, preventing other researchers from building on potentially interesting but incomplete ideas. Here are some highlights from our conversation: Neuroevolution today In the state of the art today, the algorithms have the ability to evolve variable topologies or different architectures. There are pretty sophisticated algorithms for evolving the architecture of a neural network; in other words, what’s connected to what, not just what the weight of those connections are—which is what deep learning is usually concerned with. There’s also an idea of how to encode very, very large patterns of connectivity. This is something that’s been developed independently in neuroevolution where there’s not a really analogous thing in deep learning right now. This is the idea that if you’re evolving something that’s really large, then you probably can’t afford to encode the whole thing in the DNA. In other words, if we have 100 trillion connections in our brains, our DNA does not have 100 trillion genes. In fact, it couldn’t have a 100 trillion genes. It just wouldn’t fit. That would be astronomically too high. So then, how is it that with a much, much smaller space of DNA, which is about 30,000 genes or so, three billion base pairs, how would you get enough information in there to encode something that’s 100 trillion parts? This is the issue of encoding. We’ve become sophisticated at creating artificial encodings that are basically compressed in an analogous way, where you can have a relatively short string of information to describe a very large structure that comes out—in this case, a neural network. We’ve gotten good at doing encoding and we’ve gotten good at searching more intelligently through the space of possible neural networks. We originally thought what you need to do is just breed by choosing among the best. So, you say, ‘Well, there’s some task we’re trying to do and I’ll choose among the best to create the next generation.’ We’ve learned since then that that’s actually not always a good policy. Sometimes you want to explicitly choose for diversity. In fact, that can lead to better outcomes. The myth of the objective Our book does recognize that sometimes pursuing objectives is a rational thing to do. But I think the
In this episode of the Data Show, I spoke with Ken Stanley, founding member of Uber AI Labs and associate professor at the University of Central Florida. Stanley is an AI researcher and a leading pioneer in the field of neuroevolution—a method for evolving and learning neural networks through evolutionary algorithms. In a recent survey article, Stanley went through the history of neuroevolution and listed recent developments, including its applications to reinforcement learning problems.
In this episode of the Data Show, I spoke with Robert Nishihara and Philipp Moritz, graduate students at UC Berkeley and members of RISE Lab. I wanted to get an update on Ray, an open source distributed execution framework that makes it easy for machine learning engineers and data scientists to scale reinforcement learning and other related continuous learning algorithms. Many AI applications involve an agent (for example a robot or a self-driving car) interacting with an environment. In such a scenario, an agent will need to continuously learn the right course of action to take for a specific state of the environment. What do you need in order to build large-scale continuous learning applications? You need a framework with low-latency response times, one that is able to run massive numbers of simulations quickly (agents need to be able explore states within an environment), and supports heterogeneous computation graphs. Ray is a new execution framework written in C++ that contains these key ingredients. In addition, Ray is accessible via Python (and Jupyter Notebooks), and comes with many of the standard reinforcement learning and related continuous learning algorithms that users can easily call. As Nishihara and Moritz point out, frameworks like Ray are also useful for common applications such as dialog systems, text mining, and machine translation. Here are some highlights from our conversation: Tools for reinforcement learning Ray is something we’ve been building that’s motivated by our own research in machine learning and reinforcement learning. If you look at what researchers who are interested in reinforcement learning are doing, they’re largely ignoring the existing systems out there and building their own custom frameworks or custom systems for every new application that they work on. … For reinforcement learning, you need to be able to share data very efficiently, without copying it between multiple processes on the same machine, you need to be able to avoid expensive serialization and deserialization, and you need to be able to create a task and get the result back in milliseconds instead of hundreds of milliseconds. So, there are a lot of little details that come up. … In fact, people often use MPI along with lower-level multi-processing libraries to build the communication infrastructure for their reinforcement learning applications. Scaling machine learning in dynamic environments I think right now when we think of machine learning, we often think of supervised learning. But a lot of machine learning applications are changing from making just one prediction to making sequences of decisions and taking sequences of actions in dynamic environments. The thing that’s special about reinforcement learning is it’s not just the different algorithms that are being used, but rather the different problem domain that it’s being applied to: interactive, dynamic, real-time settings bring up a lot of new challenges. … The set of algorithms actually goes even a little bit further. Some of these techniques are even useful in, for example, things like text summarization and translation. You can use these techniques that have been developed in the context of reinforcement learning to better tackle some of these more classical problems [where you have some objective function that may not be easily differentiable]. … Some of the classic applications that we have in mind when we think about reinforcement learning are things like dialogue systems, where the agent is one participant in the conversation. Or robotic control, where the agent is the robot itself and it’s trying to learn how to control its motion. … For example, we implemented the evolution algorithm described in a recent OpenAI paper in Ray. It was very easy to port to Ray, and writing it only took a couple of hours. Then we had a distributed implementation that scaled very well and we ran it on up to 1
In this episode of the Data Show, I spoke with Robert Nishihara and Philipp Moritz, graduate students at UC Berkeley and members of RISE Lab. I wanted to get an update on Ray, an open source distributed execution framework that makes it easy for machine learning engineers and data scientists to scale reinforcement learning and other related continuous learning algorithms. Many AI applications involve an agent (for example a robot or a self-driving car) interacting with an environment. In such a scenario, an agent will need to continuously learn the right course of action to take for a specific state of the environment.
In this episode of the Data Show, I spoke with Soumith Chintala, AI research engineer at Facebook. Among his many research projects, Chintala was part of the team behind DCGAN (Deep Convolutional Generative Adversarial Networks), a widely cited paper that introduced a set of neural network architectures for unsupervised learning. Our conversation centered around PyTorch, the successor to the popular Torch scientific computing framework. PyTorch is a relatively new deep learning framework that is fast becoming popular among researchers. Like Chainer, PyTorch supports dynamic computation graphs, a feature that makes it attractive to researchers and engineers who work with text and time-series. Here are some highlights from our conversation: The origins of PyTorch TensorFlow addressed one part of the problem, which is quality control and packaging. It offered a Theano style programming model, so it was a very low-level deep learning framework. … There are a multitude of front ends that are trying to cope with the fact that TensorFlow is a very low-level framework—there’s TF-slim, there’s Keras. I think there’s like 10 or 15, and just from Google there’s probably like four or five of those. On the Torch side, the philosophy has always been slightly different than Theano. I see TensorFlow as a much better Theano-style framework, and on the Torch side we had a philosophy that we want to be imperative, which means that you run your computation immediately. Debugging should be butter smooth. The user should never have trouble debugging their programs, whether they use a Python debugger or something like the GDB or something else. … Chainer was a huge inspiration. PyTorch is inspired primarily by three frameworks. Within the Torch community, certain researchers from Twitter built an auxiliary package called Autograd, and this was actually based on a package called Autograd in the Python community. Like Chainer, Autograd and Torch Autograd, all used a certain technique called tape-based automatic differentiation: that is, you have a tape recorder that records what operations you have performed and then it replays it backward to compute your gradients. This is a technique that is not used by any of the other major frameworks except PyTorch and Chainer. All of the other frameworks use what we call a static graph—that is, the user builds a graph, then they give that graph to an execution engine that is provided by the framework, and the framework executes it. It can analyze it ahead of time. These are very two different techniques. The tape-based differentiation gives you easier debuggability, and it gives you certain things that are more powerful (e.g., dynamic neural networks). The static graph-based approach gives you easier deployment to mobile, easier deployment to more exotic architectures, the ability to do compiler techniques ahead of time, and so on. Deep learning frameworks within Facebook Internally at Facebook, we have a unified strategy. We say PyTorch is used for all of research and Caffe 2 is used for all of production. This makes it easier for us to separate out which team does what and which tools do what. What we are seeing is, users first create a PyTorch model. When they are ready to deploy their model into production, they just convert it into a Caffe 2 model, then ship into either mobile or another platform. PyTorch user profiles PyTorch has gotten its biggest adoption from researchers, and it’s gotten about a moderate response from data scientists. As we expected, we did not get any adoption from product builders because PyTorch models are not easy to ship into mobile, for example. We also have people who we did not expect to come on board, like folks from OpenAI and several universities. Related resources: Building intelligent applications with deep learning and TensorFlow BigDL: Deep learning for Apache Spark MXNet: Deep learning that’s easy to implement and easy to
In this episode of the Data Show, I spoke with Soumith Chintala, AI research engineer at Facebook. Among his many research projects, Chintala was part of the team behind DCGAN (Deep Convolutional Generative Adversarial Networks), a widely cited paper that introduced a set of neural network architectures for unsupervised learning. Our conversation centered around PyTorch, the successor to the popular Torch scientific computing framework. PyTorch is a relatively new deep learning framework that is fast becoming popular among researchers. Like Chainer, PyTorch supports dynamic computation graphs, a feature that makes it attractive to researchers and engineers who work with text and time-series.
In this episode of the Data Show, I spoke with Evangelos Simoudis, co-founder of Synapse Partners and a frequent contributor to O’Reilly. He recently published a book entitled The Big Data Opportunity in Our Driverless Future, and I wanted get his thoughts on the transportation industry and the role of big data and analytics in its future. Simoudis is an entrepreneur, and he also advises and invests in many technology startups. He became interested in the automotive industry long before the current wave of autonomous vehicle startups was in the planning stages. Here are some highlights from our conversation: Understanding the automotive industry The more I started spending time with the automotive industry, the more I came to realize that, because of the autonomous vehicle technology and because of various forms of mobility services, which are stemming from new business models, the incumbent automotive industry is in significant risk of being disrupted. If you were to look at the automotive industry, the first thing that is very striking is that there’s a small number of very large companies that control a number of different labels. With GM, we talk about Chevy, we talk about Buick, we talk about Opel in Europe. There are a very small number of companies that control this trillion dollar industry. The other thing that is interesting is that these companies are responsible for designing the vehicle, manufacturing it, assembling it, post-manufacturing, and then creating demand, whereas the sale of the vehicle is done through the dealers. And they’re paying relatively little attention to what happens post-sale. So, that means there is a relatively little understanding of consumer behavior. The third observation is that the reason there are so few of these companies is because starting one is very capital intensive. And if you look at how much money, for example, a company like Tesla has been able to raise, you get a sense of what kind of capital is necessary. And the next point is that even though there is a lot of capital that’s being raised, in the end this is a relatively low margin business. Where you try to make it up is in volume. That’s why, if you look at all these corporations, they have extremely sophisticated supply chains, extremely sophisticated manufacturing lines, highly optimized, because they are working on maintaining these margins. Infrastructure for autonomous vehicles A vehicle needs to know very much what’s happening around it. So that means it needs to receive signals from roads, bridges, other vehicles. … The term people use is V2X or vehicle-to-everything communication. It will take a very long time to have the preponderance of vehicles being autonomous. So, we need infrastructure that will enable cars to safely operate in a hybrid world between autonomous vehicles and manually operated vehicles. I think the experiments that today involve just a few tens of cars will expand over the next few years. And I think the result of those experiments will give us an understanding and appreciation of the investments that we need to make and how to prioritize them, as well as the regulations that we will need to institute in order to have this type of hybrid environment operate safely. AI and big data The argument that I’m making, and this actually comes from my education on AI and my work on AI since the mid ‘80s, is that while machine learning is important, I think everybody needs to appreciate that it’s not only about machine learning. In order to bring to realization an autonomous vehicle, you need more than machine learning. And, of course, within machine learning we have neural network learning and particularly deep learning, and these are very important areas. But people need to realize that an autonomous vehicle requires the ability to plan, requires the ability to reason, to represent knowledge, to search. All of these are components of AI. What I’m ho
In this episode of the Data Show, I spoke with Evangelos Simoudis, co-founder of Synapse Partners and a frequent contributor to O’Reilly. He recently published a book entitled The Big Data Opportunity in Our Driverless Future, and I wanted get his thoughts on the transportation industry and the role of big data and analytics in its future. Simoudis is an entrepreneur, and he also advises and invests in many technology startups. He became interested in the automotive industry long before the current wave of autonomous vehicle startups was in the planning stages.