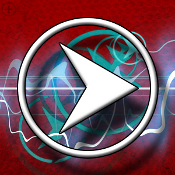
O'Reilly Data Show Podcast
Summary: The O'Reilly Data Show Podcast explores the opportunities and techniques driving big data, data science, and AI.
- Visit Website
- RSS
- Artist: O'Reilly Media
- Copyright: © 2020 O'Reilly Media
Podcasts:
In this episode of the Data Show, I spoke with Kartik Hosanagar, professor of technology and digital business, and professor of marketing at The Wharton School of the University of Pennsylvania. Hosanagar is also the author of a newly released book, A Human’s Guide to Machine Intelligence, an interesting tour through the recent evolution of AI applications that draws from his extensive experience at the intersection of business and technology. We had a great conversation spanning many topics, including: The types of unanticipated consequences of which algorithm designers should be aware. The predictability-resilience paradox: as systems become more intelligent and dynamic, they also become more unpredictable, so there are trade-offs algorithms designers must face. Managing risk in machine learning: AI application designers need to weigh considerations such as fairness, security, privacy, explainability, safety, and reliability. A bill of rights for humans impacted by the growing power and sophistication of algorithms. Some best practices for bringing AI into the enterprise. Related resources: “Managing risk in machine learning”: considerations for a world where ML models are becoming mission critical Francesca Lazzeri and Jaya Mathew on “Lessons learned while helping enterprises adopt machine learning” Jerry Overton on “Teaching and implementing data science and AI in the enterprise” Kris Hammond on “Bringing AI into the enterprise” Jacob Ward on “How social science research can inform the design of AI systems” “Overcoming barriers to AI adoption” Sharad Goel and Sam Corbett-Davies on “Why it’s hard to design fair machine learning models”
In this episode of the Data Show, I spoke with Kartik Hosanagar, professor of technology and digital business, and professor of marketing at The Wharton School of the University of Pennsylvania. Hosanagar is also the author of a newly released book, A Human’s Guide to Machine Intelligence, an interesting tour through the recent evolution of AI applications that draws from his extensive experience at the intersection of business and technology.
In this episode of the Data Show, I spoke with P.W. Singer, strategist and senior fellow at the New America Foundation, and a contributing editor at Popular Science. He is co-author of an excellent new book, LikeWar: The Weaponization of Social Media, which explores how social media has changed war, politics, and business. The book is essential reading for anyone interested in how social media has become an important new battlefield in a diverse set of domains and settings. We had a great conversation spanning many topics, including: In light of the 10th anniversary of his earlier book Wired for War, we talked about progress in robotics over the past decade. The challenge posed by the fact that social networks reward virality, not veracity. How the internet has emerged as an important new battlefield. How this new online battlefield changes how conflicts are fought and unfold. How many of the ideas and techniques covered in LikeWar are trickling down from nation-state actors influencing global events, to consulting companies offering services that companies and individuals can use. Here are some highlights from our conversation: LikeWar We spent five years tracking how social media was being used all around the world. … We looked at everything from how was it being used by militaries, by terrorist groups, by politicians, by teenagers—you name it. The finding of this project is sort of a two-fold play on words. The first is, if you think of cyberwar as the hacking of networks, LikeWar is its twin. It’s the hacking of people on the networks by driving ideas viral through a mix of likes and lies. … Social media began as a space for fun, for entertainment. It then became a communication space. It became a marketplace. It’s also turned it into a kind of battle space. It’s simultaneously all of these things at once, and you can see, for example, Russian information warriors who are using digital marketing techniques and teenage jokes to influence the outcomes of elections. A different example would be ISIS’ top recruiter, Junaid Hussain, mimicking how Taylor Swift built her fan army. A common set of tactics The second finding of the project was that when you look across all these wildly diverse actors, groups, and organizations, they turned out to be using very similar tactics, very similar approaches. To put it a different way: it’s a mode of conflict. There’s ways of “winning” that all the different groups are realizing. More importantly, the groups that understand these new rules of the game are the ones that are winning their online wars and having a real effect, whether that real effect is winning a political campaign, winning a corporate marketing campaign, winning a campaign to become a celebrity, or to become the most popular kid in school. Or “winning” might be to do the opposite—to sabotage someone else’s campaign to become a leading political candidate. Related resources: Siwei Lyu on “The technical, societal, and cultural challenges that come with the rise of fake media” Supasorn Suwajanakorn on “Building artificial people: Endless possibilities and the dark side” Guillaume Chaslot on “The importance of transparency and user control in machine learning” “Overcoming barriers to AI adoption” Alon Kaufman on “Machine learning on encrypted data” Sharad Goel and Sam Corbett-Davies on “Why it’s hard to design fair machine learning models”
In this episode of the Data Show, I spoke with P.W. Singer, strategist and senior fellow at the New America Foundation, and a contributing editor at Popular Science. He is co-author of an excellent new book, LikeWar: The Weaponization of Social Media, which explores how social media has changed war, politics, and business. The book is essential reading for anyone interested in how social media has become an important new battlefield in a diverse set of domains and settings.
In this episode of the Data Show, I spoke with Siwei Lyu, associate professor of computer science at the University at Albany, State University of New York. Lyu is a leading expert in digital media forensics, a field of research into tools and techniques for analyzing the authenticity of media files. Over the past year, there have been many stories written about the rise of tools for creating fake media (mainly images, video, audio files). Researchers in digital image forensics haven’t exactly been standing still, though. As Lyu notes, advances in machine learning and deep learning have also found a receptive audience among the forensics community. We had a great conversation spanning many topics including: The many indicators used by forensic experts and forgery detection systems Balancing “open” research with risks that come with it—including “tipping off” adversaries State-of-the-art detection tools today, and what the research community and funding agencies are working on over the next few years. Technical, societal, and cultural challenges that come with the rise of fake media. Here are some highlights from our conversation: Imbalance between digital forensics researchers and forgers In theory, it looks difficult to synthesize media. This is true, but on the other hand, there are factors to consider on the side of the forgers. The first is the fact that most people working in forensics, like myself, usually just write a paper and publish it. So, the details of our detection algorithm becomes available immediately. On the other hand, people making fake media are usually secretive; they don’t usually publish the details of their algorithms. So, there’s a kind of imbalance between the information on the forensic side and the forgery side. The other issue is user habit. The fact that even if some of the fakes are very low quality, a typical user checks it just for a second; sees something interesting, exciting, sensational; and helps distribute it without actually checking the authenticity. This actually helps fake media to broadcast very, very fast. Even though we have algorithms to detect fake media, these tools are probably not fast enough to actually stop the trap. … Then there are the actual incentives for this kind of work. For forensics, even if we have the tools and the time to catch a piece of fake media, we don’t get anything. But for people actually making the fake media, there is more financial or other forms of incentive to do that. Related resources: Supasorn Suwajanakorn on “Building artificial people: Endless possibilities and the dark side” Alyosha Efros on “Using computer vision to understand big visual data” “Overcoming barriers to AI adoption” “What is neural architecture search?” Alon Kaufman on “Machine learning on encrypted data” Sharad Goel and Sam Corbett-Davies on “Why it’s hard to design fair machine learning models”
In this episode of the Data Show, I spoke with Siwei Lyu, associate professor of computer science at the University at Albany, State University of New York. Lyu is a leading expert in digital media forensics, a field of research into tools and techniques for analyzing the authenticity of media files. Over the past year, there have been many stories written about the rise of tools for creating fake media (mainly images, video, audio files). Researchers in digital image forensics haven’t exactly been standing still, though. As Lyu notes, advances in machine learning and deep learning have also found a receptive audience among the forensics community.
In this episode of the Data Show, I spoke with Maryam Jahanshahi, research scientist at TapRecruit, a startup that uses machine learning and analytics to help companies recruit more effectively. In an upcoming survey, we found that a “skills gap” or “lack of skilled people” was one of the main bottlenecks holding back adoption of AI technologies. Many companies are exploring a variety of internal and external programs to train staff on new tools and processes. The other route is to hire new talent. But recent reports suggest that demand for data professionals is strong and competition for experienced talent is fierce. Jahanshahi and her team are building natural language and statistical tools that can help companies improve their ability to attract and retain talent across many key areas. Here are some highlights from our conversation: Optimal job titles The conventional wisdom in our field has always been that you want to optimize for “the number of good candidates” divided by “the number of total candidates.” … The thinking is that one of the ways in which you get a good signal-to-noise ratio is if you advertise for a more senior role. … In fact, we found the number of qualified applicants was lower for the senior data scientist role. … We saw from some of our behavioral experiments that people were feeling like that was too senior a role for them to apply to. What we would call the “confidence gap” was kicking in at that point. It’s a pretty well-known phenomena that there are different groups of the population that are less confident. This has been best characterized in terms of gender. It’s the idea that most women only apply for jobs when they meet 100% of the qualifications versus most men will apply even with 60% of the qualifications. That was actually manifesting. Highlighting benefits We saw a lot of big companies that would offer 401(k), that would offer health insurance or family leave, but wouldn’t mention those benefits in the job descriptions. This had an impact on how candidates perceived these companies. Even though it’s implied that Coca-Cola is probably going to give you 401(k) and health insurance, not mentioning it changes the way you think of that job. … So, don’t forget the things that really should be there. Even the boring stuff really matters for most candidates. You’d think it would only matter for older candidates, but, actually, millennials and everyone in every age group are very concerned about these things because it’s not specifically about the 401(k) plan; it’s about what it implies in terms of the company—that the company is going to take care of you, is going to give you leave, is going to provide a good workplace. Improving diversity We found the best way to deal with representation at the end of the process is actually to deal with representation early in the process. What I mean by that is having a robust or a healthy candidate pool at the start of the process. We found for data scientist roles, that was about having 100 candidates apply for your job. … If we’re not getting to the point where we can attract 100 applicants, we’ll take a look at that job description. We’ll see what’s wrong with it and what could be turning off candidates; it could be that you’re not syndicating the job description well, it’s not getting into search results, or it could be that it’s actually turning off a lot of people. You could be asking for too many qualifications, and that turns off a lot of people. … Sometimes it involves taking a step back and taking a look at what we’re doing in this process that’s not helping us and that’s starving us of candidates. Related resources: Sharad Goel and Sam Corbett-Davies on “Why it’s hard to design fair machine learning models” “Comparing production-grade NLP libraries” “What are machine learning engineers?” “Th
In this episode of the Data Show, I spoke with Maryam Jahanshahi, research scientist at TapRecruit, a startup that uses machine learning and analytics to help companies recruit more effectively. In an upcoming survey, we found that a “skills gap” or “lack of skilled people” was one of the main bottlenecks holding back adoption of AI technologies. Many companies are exploring a variety of internal and external programs to train staff on new tools and processes. The other route is to hire new talent. But recent reports suggest that demand for data professionals is strong and competition for experienced talent is fierce. Jahanshahi and her team are building natural language and statistical tools that can help companies improve their ability to attract and retain talent across many key areas.
In this episode of the Data Show, I spoke with Andrew Burt, chief privacy officer and legal engineer at Immuta, a company building data management tools tuned for data science. Burt and cybersecurity pioneer Daniel Geer recently released a must-read white paper (“Flat Light”) that provides a great framework for how to think about information security in the age of big data and AI. They list important changes to the information landscape and offer suggestions on how to alleviate some of the new risks introduced by the rise of machine learning and AI. We discussed their new white paper, cybersecurity (Burt was previously a special advisor at the FBI), and an exciting new Strata Data tutorial that Burt will be co-teaching in March. Privacy and security are converging The end goal of privacy and the end goal of security are now totally summed up by this idea: how do we control data in an environment where that control is harder and harder to achieve, and in an environment that is harder and harder to understand? … As we see machine learning become more prominent, what’s going to be really fascinating is that, traditionally, both privacy and security are really related to different types of access. One was adversarial access in the case of security; the other is the party you’re giving the data to accessing it in a way that aligns with your expectations—that would be a traditional notion of privacy. … What we’re going to start to see is that both fields are going to be more and more worried about unintended entrances. Data lineage and data provenance One of the things we say in the paper is that as we move to a world where models and machine learning increasingly take the place of logical instruction-oriented programming, we’re going to have less and less source code, and we’re going to have more and more source data. And as that shift occurs, what then becomes most important is understanding everything we can about where that data came from, who touched it, and if its integrity has in fact been preserved. In the white paper, we talk about how, when we think about integrity in this world of machine learning and models, it does us a disservice to think about a binary state, which is the traditional way: “either data is correct or it isn’t. Either it’s been tampered with, or it hasn’t been tampered with.” And that was really the measure by which we judged whether failures had occurred. But when we’re thinking not about source code but about source data for models, we need to be moving into more of a probabilistic mode. Because when we’re thinking about data, data in itself is never going to be fully accurate. It’s only going to be representative to some degree of whatever it’s actually trying to represent. Related resources: “Managing risk in machine learning” Sharad Goel and Sam Corbett-Davies on “Why it’s hard to design fair machine learning models” Alon Kaufman on “Machine learning on encrypted data” “Managing risk in machine learning models”: Andrew Burt and Steven Touw on how companies can manage models they cannot fully explain. “We need to build machine learning tools to augment machine learning engineers” “Case studies in data ethics”
In this episode of the Data Show, I spoke with Andrew Burt, chief privacy officer and legal engineer at Immuta, a company building data management tools tuned for data science. Burt and cybersecurity pioneer Daniel Geer recently released a must-read white paper (“Flat Light”) that provides a great framework for how to think about information security in the age of big data and AI. They list important changes to the information landscape and offer suggestions on how to alleviate some of the new risks introduced by the rise of machine learning and AI. We discussed their new white paper, cybersecurity (Burt was previously a special advisor at the FBI), and an exciting new Strata Data tutorial that Burt will be co-teaching in March.
In this episode of the Data Show, I spoke with Haoyuan Li, CEO and founder of Alluxio, a startup commercializing the open source project with the same name (full disclosure: I’m an advisor to Alluxio). Our discussion focuses on the state of Alluxio (the open source project that has roots in UC Berkeley’s AMPLab), specifically emerging use cases here and in China. Given the large-scale use in China, I also wanted to get Li’s take on the state of data and AI technologies in Beijing and other parts of China. Here are some highlights from our conversation: A much needed layer between compute and storage in a world with disparate storage systems This new layer, which we call a virtual distributed file system, sits in the middle between the compute and storage layers. This new layer virtualizes data from different storage systems and presents a unified API with a global namespace for the data-driven applications to interact with all of the data in the enterprise environment. AI and machine learning applications One key reason people use an object store is that it is cheap. Per gigabyte or per terabyte, it’s cheaper than other solutions in a market,…but performance is not as good. And from that perspective, by putting open source Alluxio on top of that, that improves performance from Alluxio’s caching functionality. On top of that, in many cases, machine learning libraries cannot directly talk with object stores, and Alluxio can also serve as a translation layer. Adoption in China Things are moving very fast in that region. People are eager to adopt new technology, particularly for AI and big data. Some are users we know very quickly boosted their Alluxio deployments to hundreds of nodes or even thousands of nodes. It’s amazing to see how fast they can adapt. … Of the top 10 internet companies in China, nine are using open source Alluxio in production today. All nine of them have big data and AI use cases for Alluxio. … I also travel back and forth between these two regions quite often, and every time I go there, I see more use cases, more applications, and more innovation. Related resources: Michael Franklin on the lasting legacy of AMPLab Jason Dai on why “Companies in China are moving quickly to embrace AI technologies” Kai-Fu Lee on “China: AI superpower” Andrew Feldman on why “Specialized hardware for deep learning will unleash innovation” Greg Diamos on “How big compute is powering the deep learning rocket ship” Tim Kraska on “How machine learning will accelerate data management systems”
In this episode of the Data Show, I spoke with Haoyuan Li, CEO and founder of Alluxio, a startup commercializing the open source project with the same name (full disclosure: I’m an advisor to Alluxio). Our discussion focuses on the state of Alluxio (the open source project that has roots in UC Berkeley’s AMPLab), specifically emerging use cases here and in China. Given the large-scale use in China, I also wanted to get Li’s take on the state of data and AI technologies in Beijing and other parts of China.
For the end-of-year holiday episode of the Data Show, I turned the tables on Data Show host Ben Lorica to talk about trends in big data, machine learning, and AI, and what to look for in 2019. Lorica also showcased some highlights from our upcoming Strata Data and Artificial Intelligence conferences. Here are some highlights from our conversation: Real-world use cases for new technology If you’re someone who wants to use data, data infrastructure, data science, machine learning, and AI, we’re really at the point where there are a lot of tools for implementers and developers. They’re not necessarily doing research and development; they just want to build better products and automate workflow. I think that’s the most significant development in my mind. And then I think use case sharing also has an impact. For example, at our conferences, people are sharing how they’re using AI and ML in their businesses, so the use cases are getting better defined—particularly for some of these technologies that are relatively new to the broader data community, like deep learning. There are now use cases that touch the types of problems people normally tackle—so, things that involve structured data, for example, for time series forecasting, or recommenders. With that said, while we are in an implementation phase, I think as people who follow this space will attest, there’s still a lot of interesting things coming out of the R&D world, so still a lot of great innovation and a lot more growth in terms of how sophisticated and how easy to use these technologies will be. Addressing ML and AI bottlenecks We have a couple of surveys that we’ll release early in 2019. In one of these surveys, we asked people what the main bottleneck is in terms of adopting machine learning and AI technologies. Interestingly enough, the main bottleneck was cultural issues—people are still facing challenges in terms of convincing people within their companies to adopt these technologies. And then, of course, the next two are the ones we’re familiar with: lack of data and lack of skilled people. And then the fourth bottleneck people cited was trouble identifying business use cases. What’s interesting about that is, if you then ask people how mature their practice is and you look at the people with the most mature AI and machine learning practices, they still cite a lack of data as the main bottleneck. What that tells me is that there’s still a lot of opportunity for people to apply these technologies within their companies, but there’s a lot of foundational work people have to do in terms of just getting data in place, getting data collected and ready for analytics. Focus on foundational technologies At the Strata Data conferences in San Francisco, London, and New York, the emphasis will be building technologies, bringing in technologies and cultural practices that will allow you to sustain analytics and machine learning in your organization. That means having all of the foundational technologies in place—data ingestion, data governance, ETL, data lineage, data science platform, metadata, store, and things like that, the various pieces of technology that will be important as you scale the practice of machine learning and AI in your company. At the Artificial Intelligence conferences, we remain focused on being the de facto gathering place for people interested in applied artificial intelligence. We will focus on servicing the most important use cases in many, many domains. That means showcasing, of course, the latest research in deep learning and other branches of machine learning, but also helping people grapple with some of the other important considerations, like privacy and security, fairness, reliability, and safety. …At both the Strata Data and Artificial Intelligence conferences, we will focus on helping people understand the capabilities of the technology, the strengths and limitations; th
For the end-of-year holiday episode of the Data Show, I turned the tables on Data Show host Ben Lorica to talk about trends in big data, machine learning, and AI, and what to look for in 2019. Lorica also showcased some highlights from our upcoming Strata Data and Artificial Intelligence conferences.
In this episode of the Data Show, I spoke with Alex Wong, associate professor at the University of Waterloo, and co-founder of DarwinAI, a startup that uses AI to address foundational challenges with deep learning in the enterprise. As the use of machine learning and analytics become more widespread, we’re beginning to see tools that enable data scientists and data engineers to scale and tackle many more problems and maintain more systems. This includes automation tools for the many stages involved in data science, including data preparation, feature engineering, model selection, and hyperparameter tuning, as well as tools for data engineering and data operations. Wong and his collaborators are building solutions for enterprises, including tools for generating efficient neural networks and for the performance analysis of networks deployed to edge devices. Here are some highlights from our conversation: Using AI to democratize deep learning Having worked in machine learning and deep learning for more than a decade, both in academia as well as industry, it really became very evident to me that there’s a significant barrier to widespread adoption. One of the main things is that it is very difficult to design, build, and explain deep neural networks. I especially wanted to meet operational requirements. The process just involves way too much guesswork, trial and error, so it’s hard to build systems that work in real-world industrial systems. One of the out-of-the-box moments we had—pretty much the only way we could actually do this—was to reinvent the way we think about building deep neural networks. Which is, can we actually leverage AI itself as a collaborative technology? Can we build something that works with people to design and build much better networks? And that led to the start of DarwinAI—our main vision is pretty much enabling deep learning for anyone, anywhere, anytime. Generative synthesis The general concept of generative synthesis is to find the best generative model that meets your particular operational requirements (which could be size, speed, accuracy, and so forth). So, the intuition behind that is that we treat it as a large constrained optimization problem where we try to identify the generative machine that will actually give you the highest performance. We have a unique way of having an interplay between a generator and an inquisitor where the generator will generate networks that the inquisitor probes and understands. Then it learns intuition about what makes a good network and what doesn’t. Related resources: Vitaly Gordon on “Building tools for enterprise data science” “What machine learning means for software development” “We need to build machine learning tools to augment machine learning engineers” Tim Kraska on “How machine learning will accelerate data management systems” “Building tools for the AI applications of tomorrow”