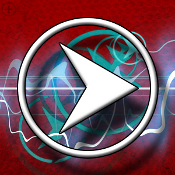
O'Reilly Data Show Podcast
Summary: The O'Reilly Data Show Podcast explores the opportunities and techniques driving big data, data science, and AI.
- Visit Website
- RSS
- Artist: O'Reilly Media
- Copyright: © 2020 O'Reilly Media
Podcasts:
In this episode of the Data Show, I spoke with Jason Dai, CTO of Big Data Technologies at Intel, and one of my co-chairs for the AI Conference in Beijing. I wanted to check in on the status of BigDL, specifically how companies have been using this deep learning library on top of Apache Spark, and discuss some newly added features. It turns out there are quite a number of companies already using BigDL in production, and we talked about some of the popular uses cases he’s encountered. We recorded this podcast while we were at the AI Conference in Beijing, so I wanted to get Dai’s thoughts on the adoption of AI technologies among Chinese companies and local/state government agencies.
In this episode of the Data Show, I spoke with Jerry Overton, senior principal and distinguished technologist at DXC Technology. I wanted the perspective of someone who works across industries and with a variety of companies. I specifically wanted to explore the current state of data science and AI within companies and public sector agencies. As much as we talk about use cases, technologies, and algorithms, there are also important issues that practitioners like Overton need to address, including privacy, security, and ethics. Overton has long been involved in teaching and mentoring new data scientists, so we also discussed some tips and best practices he shares with new members of his team. Here are some highlights from our conversation: Where most companies are in their data journey Five years ago, we had this moneyball phase where moneyball was new. This idea that you could actually get to value with data, and that data would have something to say that could help you run your business better. We’ve gone way past that now to where I think it’s pretty much a premise that if you aren’t using your data, you’re losing out on a very big competitive advantage. I think it’s pretty much a premise that data science is necessary and that you need to do something. Now, the big thing is that companies are really unsure as to what their data scientists should be doing—which areas of their business they can make smarter and how to make it smarter. … Then, you add artificial intelligence on top of this. Companies hear a lot about artificial intelligence, and they have seen some pretty cool demos—what you can do with extending domain expertise or complex planning, inferring intent, things like that. We’re entering that same phase where there are a lot of companies that are kind of skeptical as to whether or not it can actually help them. Enterprise data science. Image by Jerry Overton, used with permission. Ethics, fairness, and transparency in analytics Here are some standard things that we bring to projects. First, we have to build forensic tools to profile the algorithms being used, and then after we have a profile, we anticipate its behavior. Then we get together a diverse group and we assess the enterprise risk. … Getting people to understand that you have to handle ethics, and fairness, and bias—there are usually pretty mature programs in place for doing that. But where companies have problems is in the specific tactics for doing that. Strategies for making sure that what you put out is aligned with the ethics of the group of the company that you work in, and that’s a lot of what we help our clients with. Related resources: “Going Pro in Data Science”: Jerry Overton on what it takes to succeed as a professional data scientist “Bringing AI into the enterprise”: Kris Hammond on business applications of AI technologies and educating future AI specialists “We need to build machine learning tools to augment machine learning engineers” “Machine learning at Spotify”: Christine Hung on using data to drive digital transformation and recommenders that increase user engagement “Transforming organizations through analytics centers of excellence”: Carme Artigas on helping enterprises transform themselves with big data tools and technologies
In this episode of the Data Show, I spoke with Jerry Overton, senior principal and distinguished technologist at DXC Technology. I wanted the perspective of someone who works across industries and with a variety of companies. I specifically wanted to explore the current state of data science and AI within companies and public sector agencies. As much as we talk about use cases, technologies, and algorithms, there are also important issues that practitioners like Overton need to address, including privacy, security, and ethics. Overton has long been involved in teaching and mentoring new data scientists, so we also discussed some tips and best practices he shares with new members of his team.
In this episode of the Data Show, I spoke with Guillaume Chaslot, an ex-YouTube engineer and founder of AlgoTransparency, an organization dedicated to helping the public understand the profound impact algorithms have on our lives. We live in an age when many of our interactions with companies and services are governed by algorithms. At a time when their impact continues to grow, there are many settings where these algorithms are far from transparent. There is growing awareness about the vast amounts of data companies are collecting on their users and customers, and people are starting to demand control over their data. A similar conversation is starting to happen about algorithms—users are wanting more control over what these models optimize for and an understanding of how they work. I first came across Chaslot through a series of articles about the power and impact of YouTube on politics and society. Many of the articles I read relied on data and analysis supplied by Chaslot. We talked about his work trying to decipher how YouTube’s recommendation system works, filter bubbles, transparency in machine learning, and data privacy. Here are some highlights from our conversation: Why YouTube’s impact is less understood My theory why people completely overlooked YouTube is because on Facebook and Twitter, if one of your friends posts something strange, you’ll see it. Even if you have 1,000 friends, if one of them posts something really disturbing, you see it, so you’re more aware of the problem. Whereas on YouTube, some people binge watch some very weird things that could be propaganda, but we won’t know about it because we don’t see what other people see. So, YouTube is like a TV channel that doesn’t show the same thing to everybody and when you ask YouTube, “What did you show to other people?” YouTube says, ‘I don’t know, I don’t remember, I don’t want to tell you.’ Downsides of optimizing only for watch time When I was working on the YouTube algorithm and our goal was to optimize watch time, we were trying to make sure that the algorithm kept people online the longest. But what I realized was that we were so focused on this target of watch time that we were forgetting a lot of important things and we were seeing some very strange behavior of the algorithm. Each time we were seeing this strange behavior, we just blamed it on the user. It shows violent videos; it must be because users are violent, so it’s not our fault; the algorithm is just a mirror of human society. But if I believe the algorithm is a mirror of human society, I think it’s also not a flat mirror; it’s a mirror that emphasizes some aspects of life and makes some other aspects overlooked. … The algorithm that is behind YouTube and the Facebook news feeds are very complex, deep learning systems that will take a lot into account, including user sessions, what they’ve watched. It will try to find the right content to show to users to get them to stay online the longest and interact as much as possible with the content. So, this can seem neutral at first, but it might not be neutral. For instance, if you have content that says ‘The media is lying,’ whether it’s on Facebook or on YouTube, what will happen is that this content will naturally, if it manages to convince the user that the media is lying, the content will be very efficient at keeping the user online because the user won’t go to other media and will spend more time on YouTube and more time on Facebook. … In my personal opinion, the current goal of maximizing watch time means that any content that is really good at captivating your attention for a long time will perform really well. This means extreme content will actually perform really well. But say you had another goal—for instance, the goal to maximize likes and dislikes, or another system of rating like when you would be asked some question like, ‘Did yo
In this episode of the Data Show, I spoke with Guillaume Chaslot, an ex-YouTube engineer and founder of AlgoTransparency, an organization dedicated to helping the public understand the profound impact algorithms have on our lives. We live in an age when many of our interactions with companies and services are governed by algorithms. At a time when their impact continues to grow, there are many settings where these algorithms are far from transparent. There is growing awareness about the vast amounts of data companies are collecting on their users and customers, and people are starting to demand control over their data. A similar conversation is starting to happen about algorithms—users are wanting more control over what these models optimize for and an understanding of how they work. I first came across Chaslot through a series of articles about the power and impact of YouTube on politics and society. Many of the articles I read relied on data and analysis supplied by Chaslot. We talked about his work trying to decipher how YouTube’s recommendation system works, filter bubbles, transparency in machine learning, and data privacy.
In this episode of the Data Show, I spoke with Jesse Anderson, managing director of the Big Data Institute, and my colleague Paco Nathan, who recently became co-chair of Jupytercon. This conversation grew out of a recent email thread the three of us had on machine learning engineers, a new job role that LinkedIn recently pegged as the fastest growing job in the U.S. In our email discussion, there was some disagreement on whether such a specialized job role/title was needed in the first place. As Eric Colson pointed out in his beautiful keynote at Strata Data San Jose, when done too soon, creating specialized roles can slow down your data team. We recorded this conversation at Strata San Jose, while Anderson was in the middle of teaching his very popular two-day training course on real-time systems. We closed the conversation with Anderson’s take on Apache Pulsar, a very impressive new messaging system that is starting to gain fans among data engineers. Here are some highlights from our conversation: Why we need machine learning engineers Jesse Anderson: (2:09) One of the issues I’m seeing as I work with teams is that they’re trying to operationalize machine learning models, and the data scientists are not the one to productionize these. They simply don’t have the engineering skills. Conversely, the data engineers don’t have the skills to operationalize this either. So, we’re seeing this kind of gap in between the data science and the data engineering, and the gap I’m seeing and the way I’m seeing it being filled, is through a machine learning engineer. … I disagree with Paco that generalization is the way to go. I think it’s hyper-specialization, actually. This is coming from my experience having taught a lot of enterprises. At a startup, I would say that super-specialization is probably not going to be as possible, but at an enterprise, you are going to have to have a team that specializes in big data, and that is a part from a team, even a software engineering team, that doesn’t work with data. Putting Apache Pulsar on the radar of data engineers Key features of Apache Pulsar. Image by Karthik Ramasamy, used with permission. Jesse Anderson: (23:30) A lot of my time, since I’m really teaching data engineering is spent on data integration and data ingestion. How do we move this data around efficiently? For a lot of that time Kafka was really the only open source game in town for that. But now there’s another technology called Apache Pulsar. I’ve spent a decent amount of time actually going through Pulsar and there are some things that I see in it that Kafka will either have difficulty doing or won’t be able to do. … Apache Pulsar separates pub-sub from storage. When I first read about that, I didn’t quite get it. I didn’t quite see, why is this so important or why this is so interesting. It’s because you can individually scale your pub-sub and your storage resources independently. Now you’ve got something. Now you can say, “Well, we originally decided I wanted to store data for seven days. All right, let’s spin up some more bookkeeper processes and now we can store fourteen days, now we can store twenty one days.” I think that’s going to be a pretty interesting addition there. Where the other side of that, the corollary to that is, “Okay, we’re hitting Black Friday and we don’t have so much more data coming through as we have way more consumption and have way more things hitting our pub-sub. We could spin up more pub-sub with that.” This separation is actually allowing some interesting use cases. Related resources: “What are machine learning engineers?” “We need to build machine learning tools to augment machine learning engineers” “Differentiating via data science”: Eric Colson explains why companies must now think very differently abou
In this episode of the Data Show, I spoke with Jesse Anderson, managing director of the Big Data Institute, and my colleague Paco Nathan, who recently became co-chair of Jupytercon. This conversation grew out of a recent email thread the three of us had on machine learning engineers, a new job role that LinkedIn recently pegged as the fastest growing job in the U.S. In our email discussion, there was some disagreement on whether such a specialized job role/title was needed in the first place. As Eric Colson pointed out in his beautiful keynote at Strata Data San Jose, when done too soon, creating specialized roles can slow down your data team.
In this episode of the Data Show, I spoke with Ameet Talwalkar, assistant professor of machine learning at CMU and co-founder of Determined AI. He was an early and key contributor to Spark MLlib and a member of AMPLab. Most recently, he helped conceive and organize the first edition of SysML, a new academic conference at the intersection of systems and machine learning (ML). We discussed using and deploying deep learning at scale. This is an empirical era for machine learning, and, as I noted in an earlier article, as successful as deep learning has been, our level of understanding of why it works so well is still lacking. In practice, machine learning engineers need to explore and experiment using different architectures and hyperparameters before they settle on a model that works for their specific use case. Training a single model usually involves big (labeled) data and big models; as such, exploring the space of possible model architectures and parameters can take days, weeks, or even months. Talwalkar has spent the last few years grappling with this problem as an academic researcher and as an entrepreneur. In this episode, he describes some of his related work on hyperparameter tuning, systems, and more. Here are some highlights from our conversation: Deep learning I would say that you hear a lot about the modeling of problems associated with deep learning. How do I frame my problem as a machine learning problem? How do I pick my architecture? How do I debug things when things go wrong? … What we’ve seen in practice is that, maybe somewhat surprisingly, the biggest challenges that ML engineers face actually are due to the lack of tools and software for deep learning. These problems are sort of like hybrid systems/ML problems. Very similar to the sorts of research that came out of the AMPLab. … Things like TensorFlow and Keras, and a lot of those other platforms that you mentioned, are great and they’re a great step forward. They’re really good at abstracting low-level details of a particular learning architecture. In five lines, you can describe how your architecture looks and then you can also specify what algorithms you want to use for training. There are a lot of other systems challenges associated with actually going end to end, from data to a deployed model. The existing software solutions don’t really tackle a big set of these challenges. For example, regardless of the software you’re using, it takes days to weeks to train a deep learning model. There’s real open challenges of how to best use parallel and distributed computing both to train a particular model and in the context of tuning hyperparameters of different models. We also found out the vast majority of organizations that we’ve spoken to in the last year or so who are using deep learning for what I’d call mission-critical problems, are actually doing it with on-premise hardware. Managing this hardware is a huge challenge and something that folks like me, if I’m working at a company with machine learning engineers, have to figure out for themselves. It’s kind of a mismatch between their interests and their skills, but it’s something they have to take care of. Understanding distributed training To give a little bit more background, the idea behind this work started about four years ago. There was no deep learning in Spark MLlib at the time. We were trying to figure out how to perform distributed training of deep learning in Spark. Before actually getting our hands really dirty and trying to actually implement anything we wanted to just do some back-of-the-envelope calculations to see what speed-ups you could hope to get. … The two main ingredients here are just computation and communication. … We wanted to understand this landscape of distributed training, and, using Paleo, we’ve been able to get a good sense of this landscape without actually running experiments. The i
In this episode of the Data Show, I spoke with Ameet Talwalkar, assistant professor of machine learning at CMU and co-founder of Determined AI. He was an early and key contributor to Spark MLlib and a member of AMPLab. Most recently, he helped conceive and organize the first edition of SysML, a new academic conference at the intersection of systems and machine learning (ML). We discussed using and deploying deep learning at scale. This is an empirical era for machine learning, and, as I noted in an earlier article, as successful as deep learning has been, our level of understanding of why it works so well is still lacking. In practice, machine learning engineers need to explore and experiment using different architectures and hyperparameters before they settle on a model that works for their specific use case. Training a single model usually involves big (labeled) data and big models; as such, exploring the space of possible model architectures and parameters can take days, weeks, or even months. Talwalkar has spent the last few years grappling with this problem as an academic researcher and as an entrepreneur. In this episode, he describes some of his related work on hyperparameter tuning, systems, and more.
In this episode of the Data Show, I spoke with Ofer Ronen, GM of Chatbase, a startup housed within Google’s Area 120. With tools for building chatbots becoming accessible, conversational interfaces are becoming more prevalent. As Ronen highlights in our conversation, chatbots are already enabling companies to automate many routine tasks (mainly in customer interaction). We are still in the early days of chatbots, but if current trends persist, we’ll see bots deployed more widely and take on more complex tasks and interactions. Gartner recently predicted that by 2021, companies will spend more on bots and chatbots than mobile app development. Like any other software application, as bots get deployed in real-world applications, companies will need tools to monitor their performance. For a single, simple chatbot, one can imagine developers manually monitoring log files for errors and problems. Things get harder as you scale to more bots and as the bots get increasingly more complex. As in the case of other machine learning applications, when companies start deploying many more chatbots, automated tools for monitoring and diagnostics become essential. The good news is relevant tools are beginning to emerge. In this episode, Ronen describes a tool he helped build: Chatbase is a chatbot analytics and optimization service that leverages machine learning research and technologies developed at Google. In essence, Chatbase lets companies focus on building and deploying the best possible chatbots. Here are some highlights from our conversation: Democratization of tools for bot developers It’s been hard to get the natural language processing to work well and to recognize all the different ways people might say the same thing. There’s been an explosion of tools that leverage machine learning and natural language processing (NLP) engines to make sense of all that’s being asked of bots. But with increased capacity and capability to process data, there’s now better third-party tools for any company to take advantage of and build a decent bot out of the box. … I see three levels of bot builders out there. There’s the non-technical kind where marketing or sales might create a prototype using a user interface—like maybe Chatfuel, which requires no programming, and create a basic experience. Or they might even create some sort of decision tree bot that is not flexible, but is good for maybe basic lead-gen experiences. But they often can’t handle type-ins. It’s often button-based. So, that’s one level, the non-technical folks. Then there are teams that have developers on staff. They’re not machine learning experts, but they’re developers that can use off-the-shelf natural language processing engines to extract meaning from messages sent by users. So, you’re extracting intents and entities and making sense of what’s coming at your bot without having to have machine learning expertise. Finally, there are teams that have the machine learning experts. They might build their own NLP engine to give them more control over how it works. But often, that’s not needed if a third-party solution can serve most of your needs. But we do see some teams like that. Popular use cases We track tens and tens of thousands of bots each month with Chatbase, and what we see is that for large companies, they often start with customer support for at least two reasons. One is that the automation can save them some money, but also because chatbots enable them to create a more effective experience for their users. In fact, there’s a survey by Salesforce and a couple other companies that found that what people want from bots is quick 24/7 answers to simple questions. We also see some lead-generation bots. Those are simpler to build and often just live on a website of a company to try to gather and qualify leads. They can, most of the time, do a decent job and do a little better than just
In this episode of the Data Show, I spoke with Ofer Ronen, GM of Chatbase, a startup housed within Google’s Area 120. With tools for building chatbots becoming accessible, conversational interfaces are becoming more prevalent. As Ronen highlights in our conversation, chatbots are already enabling companies to automate many routine tasks (mainly in customer interaction). We are still in the early days of chatbots, but if current trends persist, we’ll see bots deployed more widely and take on more complex tasks and interactions. Gartner recently predicted that by 2021, companies will spend more on bots and chatbots than mobile app development. Like any other software application, as bots get deployed in real-world applications, companies will need tools to monitor their performance. For a single, simple chatbot, one can imagine developers manually monitoring log files for errors and problems. Things get harder as you scale to more bots and as the bots get increasingly more complex. As in the case of other machine learning applications, when companies start deploying many more chatbots, automated tools for monitoring and diagnostics become essential. The good news is relevant tools are beginning to emerge. In this episode, Ronen describes a tool he helped build: Chatbase is a chatbot analytics and optimization service that leverages machine learning research and technologies developed at Google. In essence, Chatbase lets companies focus on building and deploying the best possible chatbots.
In this episode of the Data Show, I spoke with Danny Lange, VP of AI and machine learning at Unity Technologies. Lange previously led data and machine learning teams at Microsoft, Amazon, and Uber, where his teams were responsible for building data science tools used by other developers and analysts within those companies. When I first heard that he was moving to Unity, I was curious as to why he decided to join a company whose core product targets game developers. As you’ll glean from our conversation, Unity is at the forefront of some of the most exciting, practical applications of deep learning (DL) and reinforcement learning (RL). Realistic scenery and imagery are critical for modern games. GANs and related semi-supervised techniques can ease content creation by enabling artists to produce realistic images much more quickly. In a previous post, Lange described how reinforcement learning opens up the possibility of training/learning rather than programming in game development. Lange explains why simulation environments are going to be important tools for AI developers. We are still in the early days of machine intelligence, and I am looking forward to more tools that can democratize AI research (including future releases by Lange and his team at Unity). Here are some highlights from our conversation: Why reinforcement learning is so exciting I’m a huge fan of reinforcement learning. I think it has incredible potential, not just in game development but in a lot of other areas, too. … What we are doing at Unity is basically making reinforcement learning available to the masses. We have shipped open source software on GitHub called Unity ML Agents, that include the basic frameworks for people to experiment with reinforcement learning. Reinforcement learning is really creating a machine learned-driven feedback loop. Recall the example I previously wrote about, of the chicken crossing the road; yes, it gets hit thousands and thousands of times by these cars, but every time it gets hit, it learns that’s a bad thing. And every time it manages to pick up a gift package on the way over the road, that’s a good thing. Over time, it gets superhuman capabilities in crossing this road, and that is fantastic because there’s not a single line of code going into that. It’s pure simulation, and through reinforcement learning it captures a method. It learns a method to cross the road, and you can take that into many different aspects of games. There are many different methods you can train. You can add two chickens—can they collaborate to do something together? We are looking at what we call multi-agent systems, where two or more of these trained reinforcement learning-trained agents are acting together to achieve a goal. … I want a million developers to start working on this. I want a lot more innovation, and I want a lot more out-of-the-box thinking, and that is what we want by making our RL tools and platform available to our Unity community. Let me just jump to one thing here: most people think that reinforcement learning in the game world or in game-like situations is a lot about what we call ‘path finding.’ Path finding is basically for a character in a game to navigate through some situation—this is pretty well understood. There are good algorithms for that. Looking ahead, I’m actually thinking about a different set of decisions. For instance, which weapon or which tool should a character pick up and bring with them in a game? That is a much, much harder decision. It’s strategy at a higher level. Machine learning and AI at Unity If you think about where intelligence originated around us (animals and humans), it’s really originating out of surviving and thriving in a physical world. That is really the job of intelligence. You have to survive, you have to find food, you have to avoid your enemies, you have to walk falling down—so, gravity is playing a big role there. If you thi
In this episode of the Data Show, I spoke with Danny Lange, VP of AI and machine learning at Unity Technologies. Lange previously led data and machine learning teams at Microsoft, Amazon, and Uber, where his teams were responsible for building data science tools used by other developers and analysts within those companies. When I first heard that he was moving to Unity, I was curious as to why he decided to join a company whose core product targets game developers. As you’ll glean from our conversation, Unity is at the forefront of some of the most exciting, practical applications of deep learning (DL) and reinforcement learning (RL). Realistic scenery and imagery are critical for modern games. GANs and related semi-supervised techniques can ease content creation by enabling artists to produce realistic images much more quickly. In a previous post, Lange described how reinforcement learning opens up the possibility of training/learning rather than programming in game development. Lange explains why simulation environments are going to be important tools for AI developers. We are still in the early days of machine intelligence, and I am looking forward to more tools that can democratize AI research (including future releases by Lange and his team at Unity).
In this episode of the Data Show, I spoke with Leo Meyerovich, co-founder and CEO of Graphistry. Graphs have always been part of the big data revolution (think of the large graphs generated by the early social media startups). In recent months, I’ve come across companies releasing and using new tools for creating, storing, and (most importantly) analyzing large graphs. There are many problems and use cases that lend themselves naturally to graphs, and recent advances in hardware and software building blocks have made large-scale analytics possible. Starting with his work as a graduate student at UC Berkeley, Meyerovich has pioneered the combination of hardware and software acceleration to create truly interactive environments for visualizing large amounts of data. Graphistry has built a suite of tools that enables analysts to wade through large data sets and investigate business and security incidents. The company is currently focused on the security domain—where it turns out that graph representations of data are things security analysts are quite familiar with. Here are some highlights from our conversation: Graphs as the front end for machine learning They’re really flexible. First of all, there’s a pure analytic reason in that there are certain types of queries that one could do efficiently with a graph database. If you needed do a bunch of joins, graphs are really great at that. … Companies want to get into stuff like 360-degree views of things; they want to understand correlations to actually explain what’s going on at a more intelligent level. … I think that’s where graphs really start to shine. Because companies deal with pretty heterogeneous data, and a graph ends up being a really easy way to deal with that. A lot of questions are basically, “What’s nearby?”—almost like your nearest neighbor type of stuff; the graph becomes, both at the query level and at the visual level, very interpretable. I now have a hypothesis about graphs as being the front end and the UI for machine learning, but that might be a topic for another day. Graph applications and correlation services If we’re talking about investigating a financial crime, you’ve got a transaction or user. … For example, if the user has multiple names but all the names are using the same address, you’re going to want to see that relationship. … In security, where a lot of my mind is today, there is something called Kill Chain, where if you think of any bad incident, there’s probably a sequence of events around it, that led up to it. … You can map out that Kill Chain. So, in a sense, a lot of the reason Graphistry uses graphs is so we can let people see that sort of progression of events and reason about it. … When people are using the graphs, especially in an enterprise setting, I think there’s a process change that’s happening if you’re building an enterprise data lake type of system. … It’s great if you can get individual alerts and create cases and investigations around individual alerts. But increasingly, you want a higher-level thing. … Instead of looking at individual alerts or individual events, you really want to think of incidents—an incident is basically a collection of alerts. For example, maybe there’s some fraud going on; if somebody figured out how to do fraud once, they’re probably going to try doing it multiple times. So, you don’t want to be playing whack-a-mole on little symptoms in each individual case; you want to get that full group incident. A graph becomes basically a way to create a real correlation service. (Full disclosure: I’m an advisor to Graphistry.) Related resources: “Graph databases are powering mission-critical applications”: Emil Eifrem on popular applications of graph technologies “Semi-supervised, unsupervised, and adaptive algorithms for large-scale time series”: Ira Cohen on developi
In this episode of the Data Show, I spoke with Leo Meyerovich, co-founder and CEO of Graphistry. Graphs have always been part of the big data revolution (think of the large graphs generated by the early social media startups). In recent months, I’ve come across companies releasing and using new tools for creating, storing, and (most importantly) analyzing large graphs. There are many problems and use cases that lend themselves naturally to graphs, and recent advances in hardware and software building blocks have made large-scale analytics possible. Starting with his work as a graduate student at UC Berkeley, Meyerovich has pioneered the combination of hardware and software acceleration to create truly interactive environments for visualizing large amounts of data. Graphistry has built a suite of tools that enables analysts to wade through large data sets and investigate business and security incidents. The company is currently focused on the security domain—where it turns out that graph representations of data are things security analysts are quite familiar with.