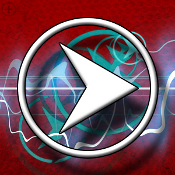
O'Reilly Data Show Podcast
Summary: The O'Reilly Data Show Podcast explores the opportunities and techniques driving big data, data science, and AI.
- Visit Website
- RSS
- Artist: O'Reilly Media
- Copyright: © 2020 O'Reilly Media
Podcasts:
In this episode of the Data Show, I spoke with Alex Wong, associate professor at the University of Waterloo, and co-founder of DarwinAI, a startup that uses AI to address foundational challenges with deep learning in the enterprise. As the use of machine learning and analytics become more widespread, we’re beginning to see tools that enable data scientists and data engineers to scale and tackle many more problems and maintain more systems. This includes automation tools for the many stages involved in data science, including data preparation, feature engineering, model selection, and hyperparameter tuning, as well as tools for data engineering and data operations. Wong and his collaborators are building solutions for enterprises, including tools for generating efficient neural networks and for the performance analysis of networks deployed to edge devices.
In this episode of the Data Show, I spoke with Vitaly Gordon, VP of data science and engineering at Salesforce. As the use of machine learning becomes more widespread, we need tools that will allow data scientists to scale so they can tackle many more problems and help many more people. We need automation tools for the many stages involved in data science, including data preparation, feature engineering, model selection and hyperparameter tuning, as well as monitoring. I wanted the perspective of someone who is already faced with having to support many models in production. The proliferation of models is still a theoretical consideration for many data science teams, but Gordon and his colleagues at Salesforce already support hundreds of thousands of customers who need custom models built on custom data. They recently took their learnings public and open sourced TransmogrifAI, a library for automated machine learning for structured data, which sits on top of Apache Spark. Here are some highlights from our conversation: The need for an internal data science platform It’s more about how much commonality there is between every single data science use case—how many of the problems are redundant and repeatable. … A lot of data scientists solve problems that honestly have a lot to do with engineering, a lot to do with things that are not pure modeling. TransmogrifAI TransmogrifAI is an automated machine library for mostly structured data, and the problem that it aims to solve is that we at Salesforce have hundreds of thousands of customers. While all of them share a common set of data, the Salesforce platform itself is extremely customizable. Actually, 80% of the data inside the Salesforce platform actually sits in what we refer to as custom objects, which one can think of as custom tables in a database. … We don’t build models that are shared between customers. We always use a single customer’s data. We have hundreds of thousands of models potentially that we need to build, and because of that, we needed to automate the entire process. We just cannot throw people at the problem. We basically created TransmogrifAI to automate the entire end-to-end process for creating a model for a user and we decided to open source it a couple months ago. Related resources: “What machine learning means for software development” “We need to build machine learning tools to augment machine learning engineers” Francesca Lazzeri and Jaya Mathew on “Lessons learned while helping enterprises adopt machine learning” Tim Kraska on “How machine learning will accelerate data management systems” “Managing risk in machine learning models”: Andrew Burt and Steven Touw on how companies can manage models they cannot fully explain. “Lessons learned turning machine learning models into real products and services”
In this episode of the Data Show, I spoke with Vitaly Gordon, VP of data science and engineering at Salesforce. As the use of machine learning becomes more widespread, we need tools that will allow data scientists to scale so they can tackle many more problems and help many more people. We need automation tools for the many stages involved in data science, including data preparation, feature engineering, model selection and hyperparameter tuning, as well as monitoring. I wanted the perspective of someone who is already faced with having to support many models in production. The proliferation of models is still a theoretical consideration for many data science teams, but Gordon and his colleagues at Salesforce already support hundreds of thousands of customers who need custom models built on custom data. They recently took their learnings public and open sourced TransmogrifAI, a library for automated machine learning for structured data, which sits on top of Apache Spark.
In this episode of the Data Show, I spoke with Francesca Lazzeri, an AI and machine learning scientist at Microsoft, and her colleague Jaya Mathew, a senior data scientist at Microsoft. We conducted a couple of surveys this year—“How Companies Are Putting AI to Work Through Deep Learning” and “The State of Machine Learning Adoption in the Enterprise”—and we found that while many companies are still in the early stages of machine learning adoption, there’s considerable interest in moving forward with projects in the near future. Lazzeri and Mathew spend a considerable amount of time interacting with companies that are beginning to use machine learning and have experiences that span many different industries and applications. I wanted to learn some of the processes and tools they use when they assist companies in beginning their machine learning journeys. Here are some highlights from our conversation: Team data science process Francesca Lazzeri: The Data Science Process is a framework that we try to apply in our projects. Everything begins with a business problem, so external customers come to us with a business problem or a process they want to optimize. We work with them to translate these into realistic questions, into what we call data science questions. And then we move to the data portion: what are the different relevant data sources, is the data internal or external? After that, you try to define the data pipeline. We start with the core part of the data science process—that is, data cleaning—and proceed to feature engineering, model building, and model deployment and management. …There are also usually external agents involved. When I say external agents, I mean there are program managers and business experts who follow us during this process. These are individuals who are the data and domain experts. It’s a very interactive process because you go back and forth trying to understand if what you are building is something that really can be interesting to the business owners. What is holding back adoption of machine learning Jaya Mathew: One of the biggest bottlenecks is lack of talent within the organization. A company really needs to invest in either up-scaling their existing employee base, which tends to be expensive and they’re trying to figure out if that investment is really worth it. Or they need to try to hire, and hiring specific skill sets is difficult, as there is a talent shortage everywhere. Then, in addition to that, there’s also a little bit of hesitation because some of the AI and machine learning models are “black boxes”. … I think many governments and many organizations need to be able to explain what’s going on before they deploy a model. Related resources: Francesca Lazzeri and Jaya Mathew: “A day in the life of a data scientist: How do we train our teams to get started with AI?” Ashok Srivastava on why “The real value of data requires a holistic view of the end-to-end data pipeline” Jerry Overton on “Teaching and implementing data science and AI in the enterprise” Carme Artigas on “Transforming organizations through analytics centers of excellence” “Managing risk in machine learning models”: Andrew Burt and Steven Touw on how companies can manage models they cannot fully explain.
In this episode of the Data Show, I spoke with Francesca Lazzeri, an AI and machine learning scientist at Microsoft, and her colleague Jaya Mathew, a senior data scientist at Microsoft. We conducted a couple of surveys this year—“How Companies Are Putting AI to Work Through Deep Learning” and “The State of Machine Learning Adoption in the Enterprise”—and we found that while many companies are still in the early stages of machine learning adoption, there’s considerable interest in moving forward with projects in the near future. Lazzeri and Mathew spend a considerable amount of time interacting with companies that are beginning to use machine learning and have experiences that span many different industries and applications. I wanted to learn some of the processes and tools they use when they assist companies in beginning their machine learning journeys.
In this episode of the Data Show, I spoke with Alon Kaufman, CEO and co-founder of Duality Technologies, a startup building tools that will allow companies to apply analytics and machine learning to encrypted data. In a recent talk, I described the importance of data, various methods for estimating the value of data, and emerging tools for incentivizing data sharing across organizations. As I noted, the main motivation for improving data liquidity is the growing importance of machine learning. We’re all familiar with the importance of data security and privacy, but probably not as many people are aware of the emerging set of tools at the intersection of machine learning and security. Kaufman and his stellar roster of co-founders are doing some of the most interesting work in this area. Here are some highlights from our conversation: Running machine learning models on encrypted data Four or five years ago, techniques for running machine learning models on data while it’s encrypted were being discussed in the academic world. We did a few trials of this and although the results were fascinating, it still wasn’t practical. … There have been big breakthroughs that have led to it becoming feasible. A few years ago, it was more theoretical. Now it’s becoming feasible. This is the right time to build a company. Not only because of the technology feasibility but definitely because of the need in the market. From inference to training A classical example would be model inference. I have data; you have some predictive model. I want to consume your model. I’m not willing to share my data with you, so I’ll encrypt my data; you’ll apply your model to the encrypted data, so you’ll never see the data. I will never see your model. The result that comes out of this computation, which is encrypted as well, will be decrypted only by me, as I have the key. This means I can basically utilize your predictive insight, you can sell your model, and no one ever exchanged data or models between the parties. … The next frontier of research is doing model training with these type of technologies. We have some great results, and there are others who are starting to do and implement some things in hardware. … Some of our recent work around applying deep learning to encrypted data combines different methods. Homomorphic encryption has its pros and cons; secure multi-party computation has other advantages and disadvantages. We basically mash various methods together to derive very, very interesting results. … For example, we have applied algorithms to genomic data at scale and we obtained impressive performance. Related resources: Sharad Goel and Sam Corbett-Davies on “Why it’s hard to design fair machine learning models” Chang Liu on “How privacy-preserving techniques can lead to more robust machine learning models” “How to build analytic products in an age when data privacy has become critical” “Data collection and data markets in the age of privacy and machine learning” “What machine learning means for software development” “Lessons learned turning machine learning models into real products and services”
In this episode of the Data Show, I spoke with Alon Kaufman, CEO and co-founder of Duality Technologies, a startup building tools that will allow companies to apply analytics and machine learning to encrypted data. In a recent talk, I described the importance of data, various methods for estimating the value of data, and emerging tools for incentivizing data sharing across organizations. As I noted, the main motivation for improving data liquidity is the growing importance of machine learning. We’re all familiar with the importance of data security and privacy, but probably not as many people are aware of the emerging set of tools at the intersection of machine learning and security. Kaufman and his stellar roster of co-founders are doing some of the most interesting work in this area.
In this episode of the Data Show, I spoke with Jacob Ward, a Berggruen Fellow at Stanford University. Ward has an extensive background in journalism, mainly covering topics in science and technology, at National Geographic, Al Jazeera, Discovery Channel, BBC, Popular Science, and many other outlets. Most recently, he’s become interested in the interplay between research in psychology, decision-making, and AI systems. He’s in the process of writing a book on these topics, and was gracious enough to give an informal preview by way of this podcast conversation. Here are some highlights from our conversation: Psychology and AI I began to realize there was a disconnect between what is a totally revolutionary set of innovations coming through in psychology right now that are really just beginning to scratch the surface of how human beings make decisions; at the same time, we are beginning to automate human decision-making in a really fundamental way. I had a number of different people say, ‘Wow, what you’re describing in psychology really reminds me of this piece of AI that I’m building right now,’ to change how expectant mothers see their doctors or change how we hire somebody for a job or whatever it is. Transparency and designing systems that are fair I was talking to somebody the other day who was trying to build a loan company that was using machine learning to present loans to people. He and his company did everything they possibly could to not redline the people they were loaning to. They were trying very hard not to make unfair loans that would give preference to white people over people of color. They went to extraordinary lengths to make that happen. They cut addresses out of the process. They did all of this stuff to try to basically neutralize the process, and the machine learning model still would pick white people at a disproportionate rate over everybody else. They can’t explain why. They don’t know why that is. There’s some variable that’s mapping to race that they just don’t know about. But that sort of opacity—this is somebody explaining it to me who just happened to have been inside the company, but it’s not as if that’s on display for everybody to check out. These kinds of closed systems are picking up patterns we can’t explain, and that their creators can’t explain. They are also making really, really important decisions based on them. I think it is going to be very important to change how we inspect these systems before we begin trusting them. Anthropomorphism and complex systems In this book, I’m also trying to look at the way human beings respond to being given an answer by an automated system. There are some very well-established, psychological principles out there that can give us some sense of how people are going to respond when they are told what to do based on an algorithm. The people who study anthropomorphism, the imparting of intention and human attributes to an automated system, say there’s a really well-established pattern. When people are shown a very complex system and given some sort of exposure to that complex system, whether it gives them an answer or whatever it is, it tends to produce in human beings a level of trust in that system that doesn’t really have anything to do with reality. … The more complex the system, the more people tend to trust it. Related resources: Jacob Ward on “How AI will amplify the best and worst of humanity” Sharad Goel and Sam Corbett-Davies on “Why it’s hard to design fair machine learning models” “Managing risk in machine learning models”: Andrew Burt and Steven Touw on how companies can manage models they cannot fully explain. “We need to build machine learning tools to augment machine learning engineers” “Case studies in data ethics” “Haunted by data”: Maciej Ceglowski makes the case for adopting enforceable limits for data storage.
In this episode of the Data Show, I spoke with Jacob Ward, a Berggruen Fellow at Stanford University. Ward has an extensive background in journalism, mainly covering topics in science and technology, at National Geographic, Al Jazeera, Discovery Channel, BBC, Popular Science, and many other outlets. Most recently, he’s become interested in the interplay between research in psychology, decision-making, and AI systems. He’s in the process of writing a book on these topics, and was gracious enough to give an informal preview by way of this podcast conversation.
In this episode of the Data Show, I spoke with Sharad Goel, assistant professor at Stanford, and his student Sam Corbett-Davies. They recently wrote a survey paper, “A Critical Review of Fair Machine Learning,” where they carefully examined the standard statistical tools used to check for fairness in machine learning models. It turns out that each of the standard approaches (anti-classification, classification parity, and calibration) has limitations, and their paper is a must-read tour through recent research in designing fair algorithms. We talked about their key findings, and, most importantly, I pressed them to list a few best practices that analysts and industrial data scientists might want to consider. Here are some highlights from our conversation: Calibration and other standard metrics Sam Corbett-Davies: The problem with many of the standard metrics is that they fail to take into account how different groups might have different distributions of risk. In particular, if there are people who are very low risk or very high risk, then it can throw off these measures in a way that doesn’t actually change what the fair decision should be. … The upshot is that if you end up enforcing or trying to enforce one of these measures, if you try to equalize false positive rates, or you try to equalize some other classification parity metric, you can end up hurting both the group you’re trying to protect and any other groups for which you might be changing the policy. … A layman’s definition of calibration would be, if an algorithm gives a risk score—maybe it gives a score from one to 10, and one is very low risk and 10 is very high risk—calibration says the scores should mean the same thing for different groups (where the groups are defined based on some protected variable like gender, age, or race). We basically say in our paper that calibration is necessary for fairness, but it’s not good enough. Just because your scores are calibrated doesn’t mean you aren’t doing something funny that could be harming certain groups. The need to interrogate data Sharad Goel: One way to operationalize this is if you have a set of reasonable measures to be your label, you can see how much your algorithm changes if you use different measures. If your algorithm is changing a lot using these different measures, then you really have to worry about determining the right measure. What is the right thing to predict. If it’s the case that under a variety of reasonable measures everything looks kind of stable, maybe it’s less of an issue. This is very hard to carry out in practice, but I do think it’s one of the most important things to understand and to be aware of when designing these types of algorithms. … There are a lot of subtleties to these different types of metrics that are important to be aware of when designing these algorithms in an equitable way. … But fundamentally, these are hard problems. It’s not particularly surprising that we don’t have an algorithm to help us make all of these algorithms fair. … What is most important is that we really interrogate the data. Related resources: “Managing risk in machine learning models”: Andrew Burt and Steven Touw on how companies can manage models they cannot fully explain. “We need to build machine learning tools to augment machine learning engineers” “Case studies in data ethics” “Haunted by data”: Maciej Ceglowski makes the case for adopting enforceable limits for data storage.
In this episode of the Data Show, I spoke with Sharad Goel, assistant professor at Stanford, and his student Sam Corbett-Davies. They recently wrote a survey paper, “A Critical Review of Fair Machine Learning,” where they carefully examined the standard statistical tools used to check for fairness in machine learning models. It turns out that each of the standard approaches (anti-classification, classification parity, and calibration) has limitations, and their paper is a must-read tour through recent research in designing fair algorithms. We talked about their key findings, and, most importantly, I pressed them to list a few best practices that analysts and industrial data scientists might want to consider.
In this episode of the Data Show, I spoke with Alan Nichol, co-founder and CTO of Rasa, a startup that builds open source tools to help developers and product teams build conversational applications. About 18 months ago, there was tremendous excitement and hype surrounding chatbots, and while things have quieted lately, companies and developers continue to refine and define tools for building conversational applications. We spoke about the current state of chatbots, specifically about the types of applications developers are building today and how he sees conversational applications evolving in the near future. As I described in a recent post, workflow automation will happen in stages. With that in mind, chatbots and intelligent assistants are bound to improve as underlying algorithms, technologies, and training data get better. Here are some highlights from our conversation: Chatbots and state machines The first component is what we call natural language understanding, which typically means taking a short message that a user sends and extracting some meaning from it, which means turning it into structured data. In the case we talked about regarding the SQL database, if somebody asks, for example, ‘What was my ROI on my Facebook campaigns last month?’, the first thing you want to understand is that this is a data question and you want to assign it a label identifying it as a person, and they’re not saying hello, or goodbye, or thank you, but asking a specific question. Then you want to pick out those fields to help you create a query. … The second piece is, how do you actually know what to do next? How do you build a system that can hold a conversation that is coherent? What you realize very quickly is that it’s not enough to have one input always matched to the same output. For example, if you ask somebody a yes or no question and they say, ‘yes,’ the next thing to do, of course, depends on what the original question was. … Real conversations aren’t stateless; they have some context and they need to pay attention to the history. So, the way developers do that is build a state machine. Which means, for example, that you have a bot that can do some different things. It can talk about flights; it can talk about hotels. Then you define different states for when the person is still searching, or for when they are comparing different things, or for when they finish a booking. And then you have to define rules for how to behave for every input, for every possible state. Beyond state machines The problem is that [the state machine] approach works for building your first version, but it really restricts you to what we call “the happy parts,” which is where the user is compliant and cooperative and does everything you ask them to do. But in typical cases, you ask a person, “Do you like option A, or option B?” Then you probably build the path for the person saying, A, you build a path for the person saying B. But then you give it to real users, and they say, “No, I don’t like either of those.” Or they ask a question like, “Why is A so much more expensive than B?” Or, “Let me get back to you about that.” … They don’t scale, that’s the problem. If you’re a developer and somebody has a conversation with your bot and you realize that it did the wrong thing, now you have to go look back at your (literally) thousands or tens of thousands of rules to figure out which one crashed and which one did the wrong thing. You figure out where to inject one more rule to handle one more etiquette, and that just doesn’t scale at all. … With our dialogue library Rasa core, we give the user the ability to talk to the bot and provide feedback. So, in Rasa, the whole flow of dialogue is also controlled with machine learning. And it’s learned from real sample conversations. You talk to the system and if it does something wrong, you pro
In this episode of the Data Show, I spoke with Alan Nichol, co-founder and CTO of Rasa, a startup that builds open source tools to help developers and product teams build conversational applications. About 18 months ago, there was tremendous excitement and hype surrounding chatbots, and while things have quieted lately, companies and developers continue to refine and define tools for building conversational applications. We spoke about the current state of chatbots, specifically about the types of applications developers are building today and how he sees conversational applications evolving in the near future. As I described in a recent post, workflow automation will happen in stages. With that in mind, chatbots and intelligent assistants are bound to improve as underlying algorithms, technologies, and training data get better.
In this episode of the Data Show, I spoke with Eric Jonas, a postdoc in the new Berkeley Center for Computational Imaging. Jonas is also affiliated with UC Berkeley’s RISE Lab. It was at a RISE Lab event that he first announced Pywren, a framework that lets data enthusiasts proficient with Python run existing code at massive scale on Amazon Web Services. Jonas and his collaborators are working on a related project, NumPyWren, a system for linear algebra built on a serverless architecture. Their hope is that by lowering the barrier to large-scale (scientific) computation, we will see many more experiments and research projects from communities that have been unable to easily marshal massive compute resources. We talked about Bayesian machine learning, scientific computation, reinforcement learning, and his stint as an entrepreneur in the enterprise software space. Here are some highlights from our conversation: Pywren The real enabling technology for us was when Amazon announced the availability of AWS Lambda, their microservices framework, in 2014. Following this prompting, I went home one weekend and thought, ‘I wonder how hard it is to take an arbitrary Python function and marshal it across the wire, get it running in Lambda; I wonder how many I can get at once?’ Thus, Pywren was born. … Right now, we’re primarily focused on the entire scientific Python stack, so SciPy, NumPy, Pandas, Matplotlib, the whole ecosystem there. … One of the challenges with all of these frameworks and running these things on Lambda is that, right now, Lambda is a fairly constrained resource environment. Amazon will quite happily give you 3,000 cores in the next two seconds, but each one has a maximum runtime and a small amount of memory and a small amount of local disk. Part of the current active research thrust for Pywren is figuring out how to do more general-purpose computation within those resource limits. But right now, we mostly support everything you would encounter in your normal Python workflow—including Jupyter, NumPy, and scikit-learn. Numpywren Chris Ré has this nice quote: ‘Why is it easier to train a bidirectional LSTM with attention than it is to just compute the SVD of a giant matrix?’ One of these things is actually fantastically more complicated than the other, but right now, our linear algebra tools are just such an impediment to doing that sort of large-scale computation. We hope NumPyWren will enable this class of work for the machine learning community. The growing importance of reinforcement learning Ben Recht makes the argument that the most interesting problems in machine learning right now involve taking action based upon your intelligence. I think he’s right about this—taking action based upon past data and doing it in a way that is safe and robust and reliable and all of these sorts of things. That is very much the domain that has traditionally been occupied by fields like control theory and reinforcement learning. Reinforcement learning and Ray Ray is an excellent platform for building large-scale distributed systems, and it’s much more Python-native than Spark was. Ray also has much more of a focus on real-time performance. A lot of the things that people are interested in with Ray revolve around doing things like large-scale reinforcement learning—and it just so happens that deep reinforcement learning is something that everyone’s really excited about. Related resources: “Optimization, compressed sensing, and large-scale machine learning pipelines”: the O’Reilly Data Show Podcast featuring Ben Recht. “Notes from the first Ray meetup” “Practical applications of reinforcement learning in industry” “Building tools for the AI applications of tomorrow” “Toward the Jet Age of machine learning”
In this episode of the Data Show, I spoke with Eric Jonas, a postdoc in the new Berkeley Center for Computational Imaging. Jonas is also affiliated with UC Berkeley’s RISE Lab. It was at a RISE Lab event that he first announced Pywren, a framework that lets data enthusiasts proficient with Python run existing code at massive scale on Amazon Web Services. Jonas and his collaborators are working on a related project, NumPyWren, a system for linear algebra built on a serverless architecture. Their hope is that by lowering the barrier to large-scale (scientific) computation, we will see many more experiments and research projects from communities that have been unable to easily marshal massive compute resources. We talked about Bayesian machine learning, scientific computation, reinforcement learning, and his stint as an entrepreneur in the enterprise software space.