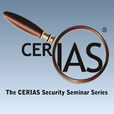
Summary: Students: This is a hybrid event. You are encouraged to attend in person in STEW 050B (G52) Space systems provide many critical functions to the military, federal agencies, and infrastructure networks. Space Policy Directive-5 Cybersecurity Principles for Space Systems describes both the cyber threat to space systems and the need for these systems to be secure and resilient against cyber-attacks. Most cyber defenses for space systems rely on the ability to detect the adversary. Reliance on detection is a risky proposition, given that anomaly and threat detection remains an open research challenge for both terrestrial and space systems. Furthermore, cyber defenses for space systems must be implemented in size, weight, and power (SWAP)-constrained, real-time operating environments that cannot tolerate increased latency and other common detrimental side-effects of cyber defenses. To overcome these challenges, we have been researching the use to moving target defenses (MTD) to protect space systems against cyber-attacks. MTDs create dynamic, uncertain environments on space systems and can be used to defeat cyber threats against these systems. Furthermore, MTDs do not require detection of an adversary to mitigate the effects of an attack. Our multi-stage-stage research approach is as follows:Development of a generalized MTD algorithm: though conceptually simple, implementation of MTD can be complex. The first research phase focused on the development of a generalized MTD algorithm that implements randomization schemes with limited impact to nominal operations and failsafe commands to re-sync devices, if needed. Application of the MTD algorithms to an exemplar: we demonstrated the MTD algorithm on real MIL-STD-1553 hardware using 4 MTD commands Functional experiments: we evaluated the reliability of the MTD algorithms and whether the use of MTD added unacceptable operational overhead. Cyber resilience experimentation and validation: we exposed the hardware and MTD to cyber-attacks to determine the effectiveness of the MTD algorithms at thwarting attacks and thereby increasing resilience to the attacks. Machine learning experiments: we used machine learning models to analyze whether the MTD algorithm introduced vulnerabilities and if the machine learning models could “crack” the MTD algorithm and predict randomization sequences. The MTD performed well in each of the experiments. Most notably, the cyber resilience experiments showed a 97% reduction in adversarial knowledge. Furthermore, small changes in the MTD algorithm substantially decreased the ability of the machine learning model to decipher randomization sequences.