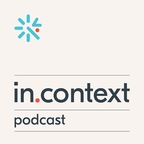
In Context
Summary: A podcast exploring the latest developments in Artificial Intelligence, within the context of the personal biographies, motivations, world views and beliefs of the leading researchers, practitioners, and entrepreneurs in the field.
- Visit Website
- RSS
- Artist: integrate.ai
Podcasts:
Could something like measurement, which seems boring on the surface, actually be the secret to getting value from machine learning? Measurement isn’t always what you think it is. Great product developers and applied machine learning practitioners are finding creative ways to incorporate it as part of their feedback loops and machine learning products. In this episode, Kathryn Hume talks with Danny Lange, the VP of and Machine Learning at Unity Technologies. In addition to discussing the role of measurement in putting reinforcement learning systems into production, they also dive into why gaming is an excellent simulation ground for the some of the most exciting developments in applied machine learning and reinforcement learning research.
In this episode of the In Context podcast, we welcome Len D’Avolio. Len is Co-Founder and CEO of Cyft, a Boston-based machine learning startup that turns healthcare organizations into learning healthcare systems. In his conversation with Kathryn Hume, they talk about what does and doesn’t work in helping companies shift from a deterministic to a probabilistic operating model through the lens of the healthcare industry.
In this episode of the In Context podcast, we're joined by Eric Colson, the Chief Algorithms Officer for Stitch Fix, an online subcription personal shopping service whose tech blog, Multithreaded, may have the coolest algorithms tour on the internet. In a fascinating discussion, he and Kathryn Hume analyze how Stitch Fix's engineering culture works, including what they value, what they look for in new hires, and how they’ve architected their platform to enable astounding success. You'll also hear about the critical role that autonomy plays in how Eric organizes his data science teams.
In this episode of the In Context podcast, we welcome Susan Etlinger, an industry analyst at Altimeter Group who focuses on data, conversational business, and ethics in the age of artificial intelligence. In their conversation, she and Kathryn Hume look at how AI is unlocking the promise of design thinking to enable amazing customer experiences, how the rapidly evolving technology landscape is changing consumer expectations around trust, and why enterprises should view ethics as a competitive differentiator rather than merely a compliance exercise. Find out how you can break these issues down and start thinking about them clearly for your business.
In our latest episode of the In Context Podcast, we welcome Clare Corthell, the Founder of Luminant Data, and Sarah Catanzaro, a Principle at Amplify Partners. Join us for a fascinating discussion about data products and where value and work occurs in machine learning pipelines. Find out about the competitive concerns that many companies have as they think about disclosing their data to third parties and the challenges and opportunities companies face as they consider entering this highly competitive space.
This episode of the In Context Podcast features a conversation with artificial intelligence researchers Randy Goebel and Osmar Zaiane, both professors at the Alberta Machine Intelligence Institute (AMII). We discuss the limitations of deep supervised learning and discuss alternatives to make it easier to understand, explain, and teach machine learning systems.
This episode features a conversation with machine learning researchers Graham Taylor (University of Guelph) and David Duvenaud (University of Toronto). We discuss how deep learning enables us to exploit the creative potential of framing tasks and phenomena as optimization problems. We cover a broad set of examples, like machine creativity, automating the design of neural network architecture, variational inference (that is, finding a good proxy representation of a tricky data set to make it usable for machine learning), and the mathematical structure behind making hard choices.
This episode of In Context features Quaid Morris, Principal Investigator at The Morris Lab at the University of Toronto and a member of the Vector Institute. Morris and his research team apply machine learning to understand biology, with projects ranging from cancer genomics to personalized medicine. The discussion includes a brief primer on genomics and DNA sequencing, insights into the challenges of working with genome data, examples of how new machine learning algorithms are changing genomics research, and thoughts on why translators are needed to realize the value machine learning can provide to science and in the enterprise.
This episode of In Context features Steve Woods, co-founder and CTO of Nudge, which applies AI to help sales professionals build deeper relationships. A veteran entrepreneur, Steve was also co-founder and CTO of Eloqua, a market leader in marketing automation eventually acquired by Oracle, and wrote Digital Body Language, a work about deciphering customer intentions online. In this wide-ranging conversation, Steve shares insights on why great sales is about theory of mind, what sales tasks should be automated and what will always remain the provenance of human creativity, why and how data science will eventually become just another form of software, and why Toronto is becoming a unique tech ecosystem.
This episode of In Context features George Smith and Daniel Dennett, both professors in the department of philosophy at Tufts University. George Smith focuses on philosophy of science and logic, with particular focus on transforming data into evidence. Co-editor of the Cambridge Companion to Newton, he's an expert on how Isaac Newton transformed notions of high-quality evidence. Daniel Dennett focuses on philosophy of mind and cognitive science, and has written multiple books on consciousness. Throughout this conversation, George and Dan share their thoughts on how theories turn data into evidence, what contributions Descartes, Newton, Hume, and Darwin made to the history of philosophy, what consciousness is and isn't, why we fall prey to anthropomorphizing machines, and how we should engage with AI systems.
This episode of In Context features Rich Sutton, a pioneer in the field of reinforcement learning, a computational approach to learning where an agent tries to maximize the total amount of reward it receives when interacting with a complex, uncertain environment. A professor in the department of Computing Science at the University of Alberta and advisor for RBC and DeepMind, Professor Sutton has devoted much of his life to AI research. His 1998 textbook, Reinforcement Learning: An Introduction, is widely used today. Throughout this conversation, Rich shares his insights on the history of reinforcement learning, current developments in temporal difference learning (a subfield of reinforcement learning), the fundamental difference between reinforcement learning and supervised learning, the importance of intuition and critical thinking in scientific research, and the parallels between learning algorithms and the big choices we make in life.