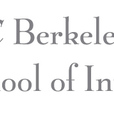
Summary: We show that Social Security numbers (SSNs) can be accurately predicted from widely available public data, such as individuals' dates and states of birth. Using only publicly available information, we observed a correlation between individuals' SSNs and their birth data, and found that for younger cohorts the correlation allows statistical inference of private SSNs, thereby heightening the risks of identity theft for millions of US residents. The inferences are made possible by the public availability of the Social Security Administration's Death Master File and the widespread accessibility of personal information from multiple sources, such as data brokers or profiles on social networking sites. Our results highlight the unexpected privacy consequences of the complex interactions among multiple data sources in modern information economies, and quantify novel privacy risks associated with information revelation in public forums. They also highlight how well-meaning policies in the area of information security can backfire, because of unanticipated interplays between policies and diverse sources of personal data.